Advancements in Protein Structure Modeling through Cryogenic Electron Microscopy
02-27-2024
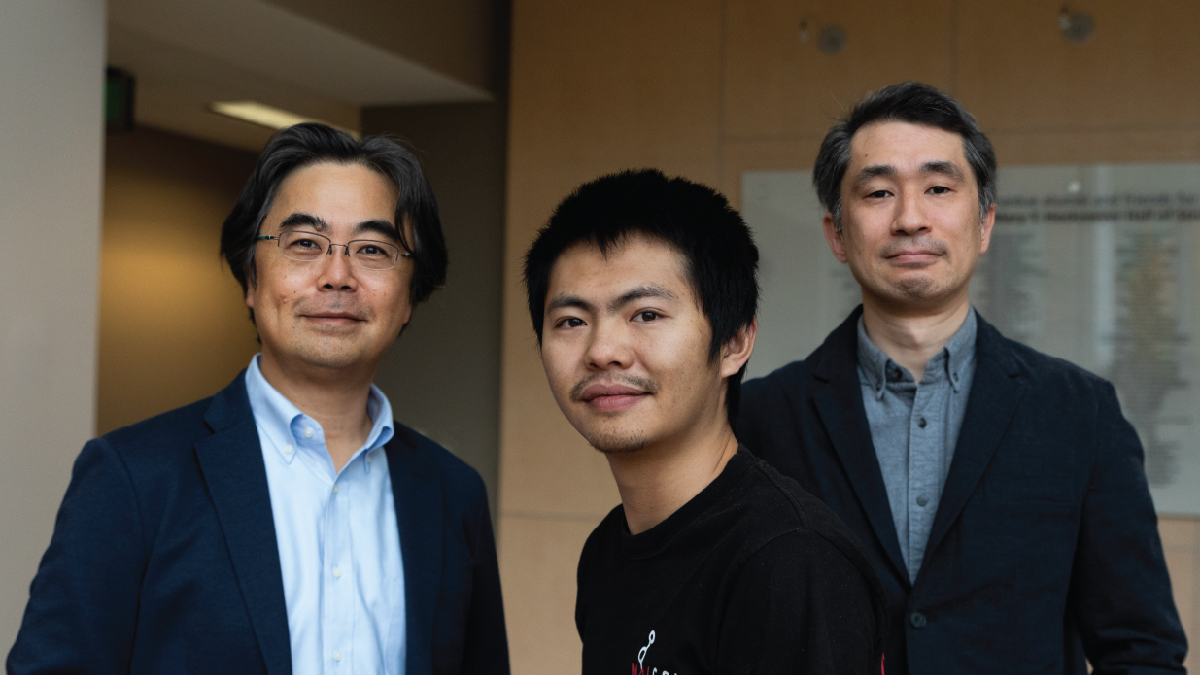
In the realm of structural biology, the ability to model protein structures accurately is paramount for understanding biological processes at the molecular level. Recent advancements in cryogenic electron microscopy (cryo-EM) have revolutionized protein structure determination, yet challenges persist in accurately tracing protein main chains from electron density maps.
In a groundbreaking study led by Daisuke Kihara, Professor of Biological Sciences and Computer Science, alongside a team of researchers including Genki Terashi, Assistant Research Scientist in Biological Sciences, Xiao Wang and Devashish Prasad, graduate students from the Department of Computer Science, and Tsukasa Nakamura, Postdoctoral Research Associate, Biological Sciences, a novel approach termed DeepMainmast has been developed to address the intricacies of protein structure modeling. This work was featured as a journal cover of the January issue of Nature Methods.
Traditionally, cryo-EM maps have provided invaluable insights into the three-dimensional structures of proteins. However, despite improvements in map resolution, accurately tracing protein main chains remains a challenge, particularly in maps determined at worse than near-atomic resolutions. This limitation spurred the development of DeepMainmast, a protein structure modeling method that integrates deep learning techniques to aid main-chain tracing from cryo-EM maps.
There are two key innovations in DeepMainmast. One is the use of deep learning, a promising AI-based approach for image processing. As cryo-EM data is an image in 3D, deep learning is effective in identifying atom positions in low resolution maps, which are otherwise not visible.
Another innovations of DeepMainmast is its integration of AlphaFold2 (AF2), a cutting-edge protein structure prediction method developed by the team. By combining the strengths of DeepMainmast's main-chain tracing with AF2's structural predictions, the researchers achieved higher accuracy in protein structure modeling than with each method alone.
The DeepMainmast protocol begins with the detection of amino acids and atoms in cryo-EM maps using a deep-learning-based method called Emap2sf. This method utilizes a neural network architecture to identify amino acid types and atom positions within the density map. Subsequently, Cα paths are traced and aligned with the protein sequence, guided by the output of Emap2sf.
Integration of AF2 models further enhances the accuracy of protein structure prediction. Fragments from AF2 models that align with DeepMainmast's main-chain trace are incorporated into the modeling process, filling gaps and refining the structure. The protocol generates multiple models that are evaluated based on various scoring metrics, including map-model fitness and amino-acid-wise model quality.
Notably, DeepMainmast outperformed existing methods in modeling single-chain proteins and multi-chain protein complexes from experimental cryo-EM maps. Its ability to accurately connect individual chains in homo-multimers sets it apart from other modeling approaches.
A similar approach, CryoREAD, was also published for DNA and RNA structure modeling for cryo-EM maps, which applies deep learning, in the same journal by the Kihara lab in late last year. With its integration of deep learning and state-of-the-art structure prediction methods, DeepMainmast represents a significant advancement in the field of structural biology, offering new insights into the intricate world of protein structures.
About the Department of Biological Sciences at Purdue University
Purdue Biological Sciences is the largest department in the Life Sciences at Purdue University. We are dedicated to pioneering scientific discoveries and transformative education at the cutting edge of innovation. From molecules to cells, from tissues to organisms, from populations to ecosystems - we bring together multiple perspectives, integrating across biological scales to advance our understanding of life and tackle the world’s most pressing challenges. Learn more at bio.purdue.edu/.
The full academic paper can be accessed in the January 2024 issue of Nature Methods.
Writer – Alisha Referda, Communications Specialist